Intelligent Design: Transform Your Website into a Sales Engine with Machine Learning
September 2014
By Kimberly Barnes
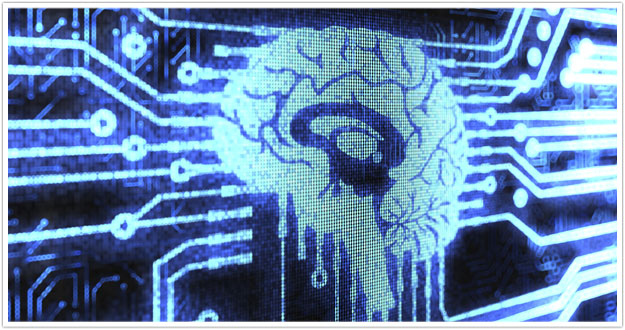
Machine learning: The phrase evokes images of computers playing chess or IBM’s Watson destroying two legendary Jeopardy! champions in a three-day tournament.
The truth is, though, machine learning is no longer a novelty; it’s now an integral part of our daily lives. Every time you receive a product recommendation from Amazon, your email server weeds out spam before it reaches your inbox or you enjoy a playlist on Pandora, you’re seeing machine learning in action.
In a nutshell, machine learning is the science of training computers to recognize data patterns and make adjustments automatically when those patterns change. While on the surface this may not sound very exciting, nothing could be further from the truth. In fact, machine learning is the key to transforming your website into a lean, mean selling machine.
Understanding machine learning in 100 words or less
Machine learning uses algorithms to build models from data; as more data is collected, the algorithms are “trained” to adapt to changes. There are two ways in which machine learning can be implemented: supervised and unsupervised.
Supervised learning algorithms are used to create models that establish relationships between types of data — the relationship between purchase data and user clickstream data, for example. Unsupervised learning uses algorithms to gain insights into customer behaviors and preferences by looking for patterns within the data.
Both of these methodologies are designed to make marketing and e-commerce more exact, more personal and more profitable.
Putting machine learning to work
Netflix, Pandora and Amazon are all familiar examples of machine learning in action. All three use recommender systems powered by complex algorithms. These systems collect data about your browsing activities, past selections and any ratings or reviews you may have provided. Then they segment you into clusters with other customers who have demonstrated similar interests or behaviors and use this data to suggest items that might appeal to you based on the browsing and purchasing habits of these other customers. You see this on Netflix as the category titled “Because you watched...” and on Amazon as “Customers who viewed this also viewed...”
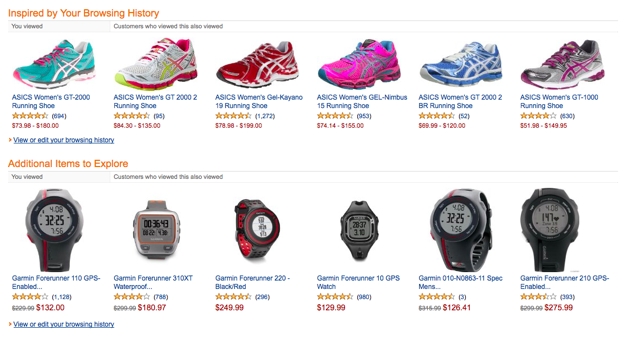
To gain a deeper understanding of how these algorithms work, let’s take a closer look at Amazon. To Amazon, you are a very long row of numbers in a massive table of data. Your row represents everything you’ve looked at, clicked on, purchased (or, equally as important, not purchased) or reviewed on the site. The other rows in this gargantuan table encompass the same thing for the millions of other customers who shop on Amazon. With every click, visit and purchase, more data is added to your row, which allows Amazon to constantly mold and shape the products it recommends to you and the special offers you receive based on an ever-evolving stream of information about you that is being collected and stored.
Another innovative example is
True Fit, a retail software start-up that is on a mission to apply data analytics to increase customer confidence in online clothing purchases while decreasing the number of returns for e-railers.
Well-known fashion retailers, including Nordstrom, Macy’s and Guess, have implemented True Fit’s algorithms on their e-commerce sites. When customers shop on these sites, they’re asked to create a profile that includes their height, weight and perhaps most importantly, the size and brand of their favorite piece of clothing.
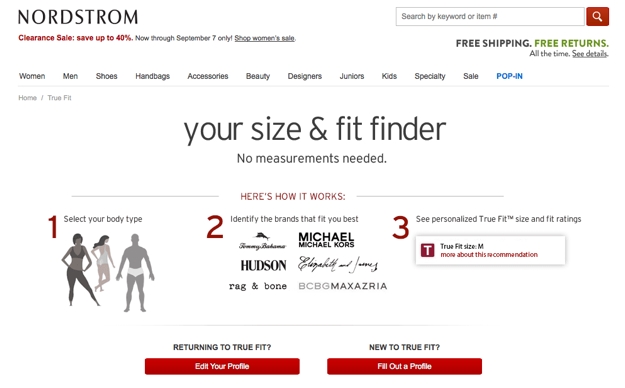
Using that data, True Fit is able to recommend the correct size for a specific brand and article of clothing. Even more importantly, as customers continue to use the True Fit system, it learns more about their personal style and preferences and steers them toward purchases they’ll be more likely to keep and enjoy rather than return.
How machine learning drives smarter marketing
You don’t need the resources of major e-commerce giants like Amazon or Netflix to take advantage of machine learning to to improve your e-commerce site and your online marketing efforts. By enhancing your existing site with systems that allow you to create a virtual marketing intelligence brain, you can create a more personalized – and therefore higher quality – shopping experience for your customers.
By establishing this type of marketing intelligence ecosystem, you can mine the data provided by customers every time they visit your site to answer vital questions that will help you fine-tune your site and your online marketing strategy – questions like these:
- How likely is a given website visitor to convert?
- What behaviors characterize customers who are likely to buy?
- What behaviors characterize customers who are likely not to buy?
- How can new visitors be identified as high-potential long-term customers?
- Which type of web traffic has the most value?
- Which products or services appeal most to a given segment of customers?
- Given the contents of a particular customer’s shopping cart, which additional products are high-potential recommendations?
- How can website visits be optimized to provide the best possible experience for each individual customer?
Making it personal
The final question in the list above is one that deserves special notice because of the staggering potential for using machine learning to create a more personalized shopping experience – one of the key drivers for increasing online sales.
Not only can the data collected via such marketing intelligence ecosystems be used to drive recommender systems, it can also be used to create personalized advertising based on market segments — or even individual profiles — that can be distributed across a variety of desktop, mobile and social platforms.
This type of advertising can be tailored to any number of personal preferences and demographic information, including age, marital status, location, lifestyle choices, typical purchases, brand preferences and so on. Ads can be focused to such a granular level that they reflect specific colors a given customer prefers, and their individual purchase drivers, such as status or cost-effectiveness.
Another exciting aspect of machine learning-based personalization is the development of individual customer profiles. You can even combine online and offline customer data to create a more complete picture of a given user. Types of data included in this profile might include online and in-store purchases, membership and activity in rewards programs, product ratings and clothing sizes. Just imagine how much more powerful your marketing efforts could be if you were armed with this level of information.
One of the most important aspects of a successful marketing intelligence ecosystem is how data mined from customer activities is combined with sound business rules in order to make smart recommendations that are well received by customers and that do not compromise their trust in your brand.
For example, most people who walk into a supermarket like bananas and will often buy some. So shouldn’t the recommender simply recommend bananas to every customer? No – because it wouldn’t help the customer, and it wouldn’t increase banana sales. So a smart supermarket recommender would always include a rule to exclude recommending bananas.
At the other end of the spectrum, the recommender shouldn’t push high-margin items just because it’s beneficial to the seller’s bottom line. It’s like going to a restaurant where the server steers you toward a particular high-dollar entree. Is it really his favorite? Or did the chef urge the staff to push the dish because it comes with a side order of premium mark-up?
To build trust, the best recommender systems strive for some degree of transparency by giving customers clues as to why a particular item was recommended and letting them adjust their profile if they don’t like the recommendations they’re receiving.
Science fact, not fiction
Machine learning can give your business a serious competitive edge by opening the door new opportunities in the marketplace. It can help you personalize and improve your customer experience dramatically and thereby drive sales and revenues. Creatives and developers alike are rapidly pioneering new and innovative ways for marketers to use machine learning — and the future of marketing built on these ideas has seemingly endless possibilities.
Kimberly Barnes is a digital marketing specialist turned freelance writer born back when Apple was called “Apple Macintosh,” floppy disks were actually floppy, and #2 pencils were the best way to rewind unraveled cassette tapes. Most days you'll find her summoning her muse while drinking a non-coffee beverage in Starbucks.